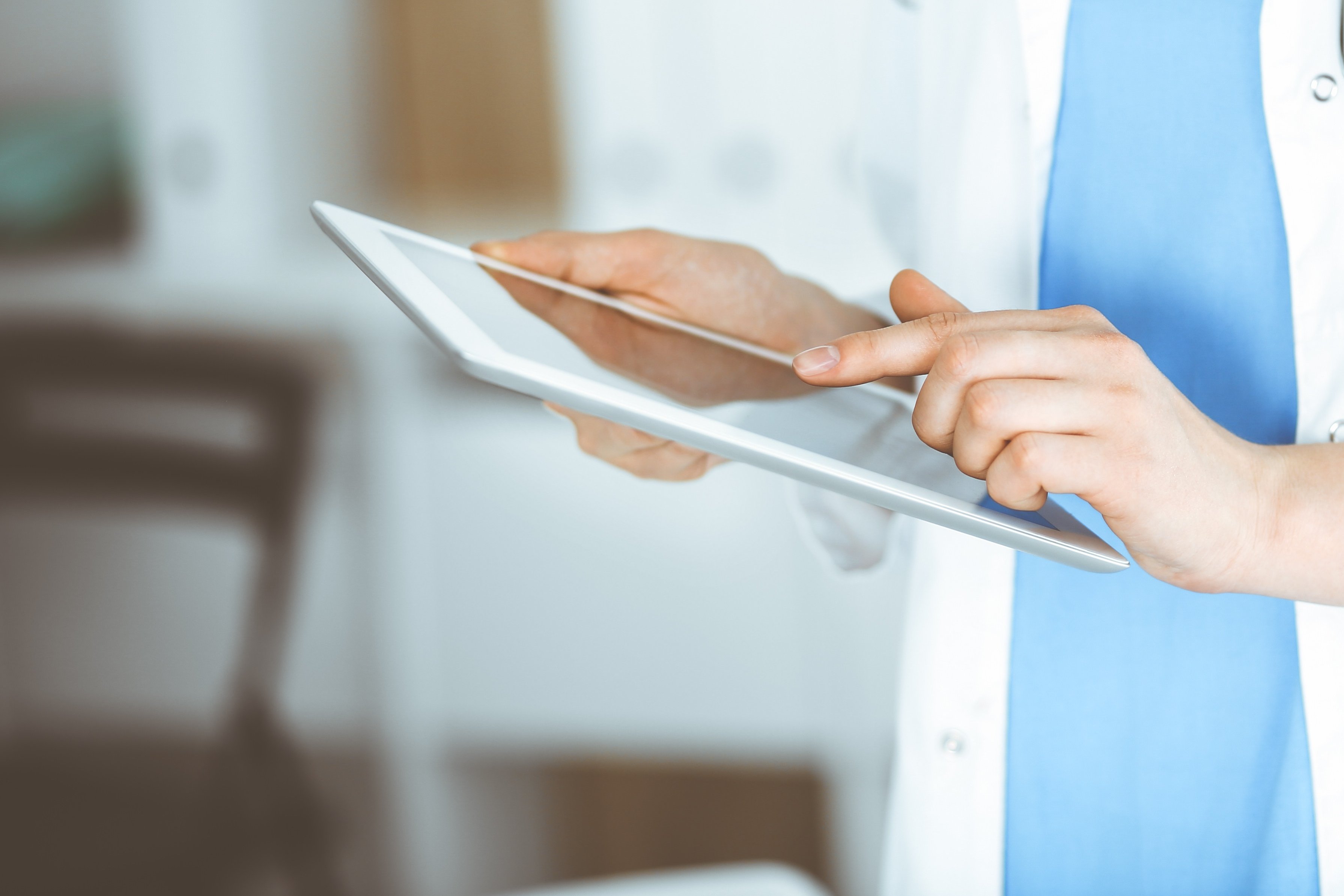
(Vienna, 27-04-2022) In everyday clinical practice, diagnosis and treatment often involve complex decisions requiring the simultaneous consideration of many different aspects and pieces of information. Using the example of personalized treatment for breast cancer, a MedUni Vienna study demonstrates the benefit of applying decision theory as a valuable extension to conventional statistics in precision medicine. The research was recently published in the "Journal of Personalized Medicine".
To investigate the potential value of decision theory in the diagnosis and treatment of breast cancer, the researchers led by study leader Wolfgang Schreiner (Institute of Biosimulation and Bioinformatics at MedUni Vienna) focused on hormone receptor status. This marker, which is relevant for selecting which breast cancer therapy to be given in each individual case, has so far been determined on the basis of immunohistochemistry. In the course of the research, the scientists have now added gene expression as another method and formulated both instruments as evidence along the lines of decision theory. This not only provides an explicit quantification of probabilities but also of the associated uncertainties and, according to the researchers, constitutes a decisive step towards improving diagnosis and therapy in precision medicine.
Evidence rather than probabilities
Classical statistics is based on properties that are "actually" present. Using the example of hormone receptor status in breast cancer, this is either receptor-positive or receptor-negative, factors that are evaluated as probabilities adding up to one (ppos+pneg=1). Decision theory, on the other hand, as already applied to self-driving cars for example, does not focus on "reality" but on the result of a measurement: positive, negative, or uncertain. These results are relativized with three weights that also add up to one: mp+mn+mu=1. The greater the uncertainty (mu), the less the two other weights of the actually meaningful diagnoses mp and mn count. "In a public election, uncertainty corresponds to non-voters," explains Wolfgang Schreiner. "Even if one of the two options is clearly predominant, for example, positive:negative =3:1, good politicians should only take that result seriously if turnout was also sufficiently high - and thus uncertainty sufficiently small." Similarly, a 'receptor-positive' result is only reliable if mu is sufficiently small: The researchers therefore conclude that this uncertainty should always be co-determined to provide evidence rather than probabilities.
Mapping decision-making behaviour as an algorithm
Another advantage of decision theory as an extension to conventional statistics is that it allows several evidence to be combined along clearly defined rules. These can be mathematically designed to either eliminate contradictions (=risky) or emphasize them (=cautious). This allows everyday clinical decision-making behaviour to be mapped in the form of decision-making algorithms - adapted to the respective situation. Decision theory also allows diagnoses to be mapped in a particularly realistic way when there are more than two options. Staying with the example of breast cancer - this could allow to assess the relevance of a histology result much more clearly than before, should it contain any fuzziness. "Overall, then, decision theory based on evidence paves the way for far-reaching improvements in diagnostics and therapy selection in precision medicine," Wolfgang Schreiner emphasizes.
Service: Journal of Personalized Medicine
Decision Theory versus Conventional Statistics for Personalized Therapy of Breast Cancer
Kenn, M. Karch, R. Cacsire Castillo-Tong, D., Singer, C.F., Kölbl, H., Schreiner W.