Christian Doppler Laboratory for Artificial Intelligence in Retina – “CD AIR”
The Lab is focused on solving socio-technical hurdles for the deployment of AI in eye care as clinical-decision support systems. This interdisciplinary research team is composed of retinal specialists, computer scientists and software engineers, developing innovative image analysis methods for precision medicine in retinal disease.
The overarching goal of the Laboratory is to enable AI-driven clinical decision support systems (CDSSs) for the effective management of retinal diseases, representing the leading causes of blindness. The CDSS would naturally be rooted in rich multi-modal imaging offered by OCT device manufacturers, but would also exploit the data available in the associated EHR. Such modern AI based CDSS in retina is expected to:
- automate tedious tasks and provide objective examination summaries,
- allow detecting pathological changes of retina early and precisely, and
- improve the clinical workflow efficiency allowing retinal specialists to examine more patients more accurately. This would constitute a groundbreaking raise of the level of healthcare quality in ophthalmology.
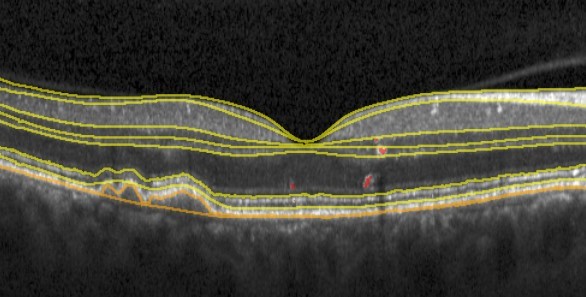
The research will advance our understanding of the most devastating blinding diseases. It will widely expand the spectrum of accessible biomarkers in the current diagnostic and therapeutic area going far beyond the previously known features and established paradigms, open the horizon for novel subclinical biomarker detection providing a novel understanding of disease mechanisms and identification of novel therapeutic targets.
Challenges ahead
With the enormous advance of AI over the past decade, nowadays capable of a human-level pattern recognition performance, uptake of deep learning models in clinical routine has nevertheless still not happened. This stems from following very unique challenges that machine learning technology is facing when being deployed in a medical setting.
- High-dimensional Data
Medical data is dense rather than big, the so called “large p, small n” problem. There is often a vast amount of multi-modal detail available about a patient while the number of available patients is limited. Such a setting poses a considerable challenge for an effective machine learning. - Data quality
Data is the fuel that powers AI, though much of real-world medical data is heterogeneous, noisy and incomplete or missing, acquired with different imaging devices and protocols. There is a large variability of expert opinions and ground truth labels are often not retrievable. Thus, machine learning methods have to handle data sparsity, and missing or incorrect values and labels. - The last mile problem, aka the final percent
In a safety-critical environment like medicine, any wrong conclusion could have a catastrophic downstream effect. Thus, it is important that AI demonstrate robustness, and is able to handle also rare, corner-case situations, which is often ignored in performance evaluations. Currently, a clinician in the loop is needed to assure the credibility of the output. - The distribution shift
It poses a fundamental limitation to machine learning abilities. As the imaging equipment changes, the models trained on retrospective data may not generalize to the newly acquired data anymore. Thus, algorithms that can withstand some extent of distribution shift are needed. - Supervision
Most of current deep learning is supervised with a significant cost and manual effort required to annotate often at the pixel-level the large amount of data needed for learning. In addition, in cases of a distribution shift, supervised learning requires extensive relabelling to regain its performance. Methodologies behind unsupervised, self-supervised and weakly-supervised approaches need to be better exploited. - Trustworthiness
AI models need to demonstrate generalizability, interpretability and reproducibility to gain trust. This places a high bar on capabilities AI needs to demonstrate. Currently, most deep learning models are essentially black-boxes, where clinicians have limited understanding of how a model comes to its decision. Further effort into providing evidence for predictions by a model is needed for clinicians to properly weigh its output. - Deployment
Use of AI in the clinic requires additional validation from socio-technical and clinical user experience aspects, to make sure the technology supports and not hinders the clinical workflows.
Despite the above challenges, we are in an exciting era at the interface of AI and retinal imaging, with ample opportunities to innovate and improve the current state of the art. This would allow to leverage the power and enourmous potential of deep learning to make a real impact in ophthalmology by further boosting retinal image analysis capabilities and enabling AI tools to find their way into the market and into the hands of the clinicians world-wide.
Research Group
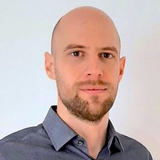
Hrvoje Bogunovic, PhD
Director of the Christian Doppler LaboratoryPhone: +43 1 40400 73419
Email: hrvoje.bogunovic@meduniwien.ac.at
Christian Doppler Laboratory for Artificial Intelligence in Retina
Department of Ophthalmology and Optometry
Medical University of Vienna
Spitalgasse 23
1090 Vienna, Austria
Office: AKH building (BT 10, 8. floor, room 08.i9.17)
Hrvoje Bogunović obtained his BSc and MSc in Computer Science from the University of Zagreb, Croatia. He obtained his PhD in 2012 from the Universitat Pompeu Fabra (UPF), Barcelona, Spain. For his thesis he worked on medical image segmentation and shape analysis applied to blood vessels in the brain. After graduation he did a postdoc at the Iowa Institute for Biomedical Imaging (IIBI), University of Iowa, US, where he started specializing in computational ophthalmic image analysis. He moved to Medical University of Vienna, Austria in 2015 to work on deep learning for retinal imaging in close collaboration with retinal specialists. As of 2018 he is a Faculty there and as of 2021 a Director of Christian Doppler Lab for Artificial Intelligence in Retina.
His general research interests are in medical image computing and machine learning for healthcare. He is particularly interested in predicting disease progression and in knowledge discovery from large clinical longitudinal imaging datasets..
Research Interests:
- Medical Image Computing
- Computational Retinal Image Analysis
- Machine Learning for Healthcare
Selected Publications:
D. Romo-Bucheli, U. Schmidt-Erfurth, H. Bogunović: “End-to-end deep learning model for predicting treatment requirements in neovascular AMD from longitudinal retinal OCT imaging”. IEEE Journal of Biomedical and Health Informatics, In Press, 2020. doi: 10.1109/JBHI.2020.3000136
U. Schmidt-Erfurth, W-D Vogl, L. Jampol, H. Bogunović: “Application of automated quantification of fluid volumes to anti-VEGF therapy of neovascular age-related macular degeneration”. Ophthalmology, In Press, 2020. doi: 10.1016/j.ophtha.2020.03.010
D. Romo-Bucheli, P. Seeböck, J.I. Orlando, B.S. Gerendas, S.M. Waldstein, U. Schmidt-Erfurth, H. Bogunović: “Reducing image variability across OCT devices with unsupervised unpaired learning for improved segmentation of retina”. Biomedical Optics Express, vol. 11(1), pp. 346-363, 2020. doi: 10.1364/BOE.379978
H. Bogunović, F. Venhuizen, S. Klimscha, S. Apostolopoulos, A. Bab-Hadiashar, U. Bagci, M.F. Beg, L. Bekalo, Q. Chen, C. Ciller, K. Gopinath,A.K. Gostar, K. Jeon, Z. Ji, S. Ho Kang, D.D. Koozekanani, D. Lu, D. Morley,K.K. Parhi, H. Suk Park, A. Rashno, M. Sarunic, S. Shaikh, J. Sivaswamy,R. Tennakoon, S. Yadav, S. De Zanet, S.M. Waldstein, B.S. Gerendas, C.Klaver, C.I. Sanchez, U. Schmidt-Erfurth: “RETOUCH-The Retinal OCT Fluid Detection and Segmentation Benchmark and Challenge”, IEEE Transactionson Medical Imaging, vol 38(8), pp. 1858-1874, 2019. doi: 10.1109/TMI.2019.2901398
P. Seebock, S.M. Waldstein, S. Klimscha, H. Bogunović, T. Schlegl, B.S.Gerendas, R. Donner, U. Schmidt-Erfurth, G. Langs: “Unsupervised Identification of Disease Marker Candidates in Retinal OCT Imaging Data”. IEEE Transaction on Medical Imaging, vol. 38(4), pp. 1037-1047, 2019. doi: 10.1109/TMI.2018.2877080
U. Schmidt-Erfurth, A. Sadeghipour, B.S. Gerendas, S.M. Waldstein, H. Bogunović: “Artificial Intelligence in Retina”. Progress in Retinal and Eye Research, vol. 67, pp. 1-29, 2018. doi: 10.1016/j.preteyeres.2018.07.004
U. Schmidt-Erfurth, S.M. Waldstein, S. Klimscha, A. Sadeghipour, X. Hu,B.S. Gerendas, A. Osborne, H. Bogunović: “Prediction of Individual Disease Conversion in Early AMD Using Artificial Intelligence”. Investigative Ophthalmology & Visual Science, vol. 59, pp. 3199-3208, 2018. doi: 10.1167/iovs.18-24106
H. Bogunović, S.M. Waldstein, T. Schlegl, G. Langs, A. Sadeghipour, X.Liu, B.S. Gerendas, A. Osborne, U. Schmidt-Erfurth. “Prediction of Anti-VEGF Treatment Requirements in Neovascular AMD Using a Machine Learning Approach”. Investigative Ophthalmology & Visual Science, vol.58, pp.3240-3248, 2017. doi: 10.1167/iovs.16-21053
H. Bogunović, A. Montuoro, M. Baratsits, M.G. Karantonis, S. M. Wald-stein, F. Schlanitz, U. Schmidt-Erfurth. “Machine Learning of the Progression of Intermediate Age-Related Macular Degeneration Based on OCT Imaging”. Investigative Ophthalmology & Visual Science, vol.58, BIO141-BIO150, 2017. doi: 10.1167/iovs.17-21789.
A. Montuoro, S.M. Waldstein, B.S. Gerendas, U. Schmidt-Erfurth, H. Bogunović: “Joint retinal layer and fluid segmentation in OCT scans of eyes with severe macular edema using unsupervised representation and auto-context”. Biomedical Optics Express, vol. 8(3), pp. 1874-1888, 2017. doi: 10.1364/BOE.8.001874
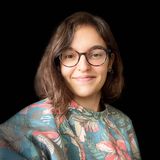
Teresa Finisterra Araújo, PhD
Computational Imaging Research | Postdoctoral ResearcherPhone: +43 1 40400 66142
Email: teresa.safinisterraaraujo@meduniwien.ac.at
Christian Doppler Laboratory for Artificial Intelligence in Retina
Department of Ophthalmology and Optometry
Medical University of Vienna
Spitalgasse 23
1090 Vienna, Austria
Office: BT72, 17. floor, room 72.17.12
Teresa Araújo received the M.Sc. degree in bioengineering and biomedical engineering from the Faculty of the Engineering, University of Porto (FEUP), Porto, Portugal, in 2016. She obtained her PhD degree in electrical and computer engineering in 2021. For her thesis she worked on diabetic retinopathy grading in color eye fundus images. Since 2014, she has collaborated as a Researcher with the Biomedical Imaging Laboratory, Center for Biomedical Engineering Research (C-BER), Institute for Systems and Computer Engineering, Technology and Science (INESC TEC), Porto. As of 2021 she has joined the Christian Doppler Laboratory for Artificial Intelligence in Retina as a postdoc researcher.
Research Interests:
- computer-aided diagnosis
- medical image analysis
- computer vision
- machine learning
- deep learning
Selected Publications:
Araújo, T., Aresta, G., Mendonça, L., Penas, S., Maia, C., et al.. DR|GRADUATE: Uncertainty-aware deep learning-based diabetic retinopathy grading in eye fundus images. Medical Image Analysis, 63:101715, 7 2020c. Doi: 10.1016/j.media.2020.101715
Aresta, G., Araújo, T., Kwok, S., Chennamse.y, S. S., Safwan, M., et al. BACH: Grand challenge on breast cancer histology images. Medical Image Analysis, 56: 122–139, 2019d. doi: 10.1016/j.media.2019.05.010
Araújo, T., Aresta, G., Mendonc¸a, L., Penas, S., Maia, C., et al. Data Augmentation for Improving Proliferative Diabetic Retinopathy Detection in Eye Fundus Images. IEEE Access, 8:182462–182474, 2020b. Doi: 10.1109/ACCESS.2020.3028960
Araújo, T., Aresta, G., Castro, E., Rouco, J., Aguiar, P., et al. Classification of breast cancer histology images using Convolutional Neural Networks. PloS ONE, 12(6):e0177544, 2017a. doi: 10.1371/journal.pone.0177544
Araújo, T., Mendonça, A. M., and Campilho, A. Parametric modeling based approach for retinal blood vessel caliber estimation in eye fundus images. PLoS ONE, 13(4):1–27, 2018b. doi: 10.1371/journal.pone.0194702
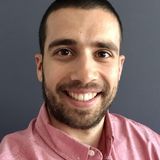
Guilherme Moreira Aresta, PhD
Computational Imaging Research | Postdoctoral ResearcherPhone: +43 1 40400 66142
Email: guilherme.moreiraaresta@meduniwien.ac.at
Christian Doppler Laboratory for Artificial Intelligence in Retina
Department of Ophthalmology and Optometry
Medical University of Vienna
Spitalgasse 23
1090 Vienna, Austria
OOffice: BT72, 17. floor, room 72.17.12
Guilherme Aresta received his M.Sc. degree in Bioengineering – Biomedical engineering from the Faculty of Engineering of University of Porto, Porto, Portugal in 2016. He later pursued his Ph.D. degree in Electrical and Computer Engineering at the same faculty, having focused on the development of deep learning approaches for medical image analysis and especially lung cancer screening. He collaborated as a researcher with the Biomedical Imaging Laboratory, Center for Biomedical Engineering Research (C-BER) of the Institute for Systems and Computer Engineering, Technology and Science (INESC-TEC) since 2014. Since 2021 he has joined the Christian Doppler Laboratory for Artificial Intelligence in Retina as a postdoc researcher.
His research interests are related to the study of machine learning, deep learning and computer vision techniques for medical image analysis and computer-aided diagnosis.
Selected Publications:
Aresta, G., Araújo, T., Kwok, S.,et al.BACH: Grand challenge on breast cancer histologyimages.Medical Image Analysis, 56:122–139, 2019c. doi: 10.1016/j.media.2019.05.010(first two authors contributed equally)
Aresta, G., Jacobs, C., Araújo, T.,et al.iW-Net: an automatic and minimalistic interactivelung nodule segmentation deep network.Scientific Reports, 9(1):1–9, 2019e. doi: 10.1038/s41598-019-48004-8
Aresta, G., Ferreira, C., Pedrosa, J.,et al.Automatic Lung Nodule Detection CombinedWith Gaze Information Improves Radiologists’ Screening Performance.IEEE journal ofbiomedical and health informatics, 24(10):2894–2901, 2020. doi: 10.1109/JBHI.2020.2976150
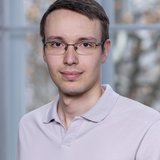
Dmitrii Lachinov, MSc
Computational Imaging Research | PhD StudentPhone: +43 1 40400 66143
Email: dmitrii.lachinov@meduniwien.ac.at
Christian Doppler Laboratory for Artificial Intelligence in Retina
Department of Ophthalmology and Optometry
Medical University of Vienna
Spitalgasse 23
1090 Vienna, Austria
Office: BT72, 17. floor, room 72.17.13
Dmitrii Lachinov received his BSc and MSc in Computer Science from Lobachevsky State University of Nizhny Novogrod, Russia. During his masters, Dmitrii was an exchange student at Sungkyunkwan University, South Korea. During his studies, Dmitrii was working on heart vessel segmentation and image registration. He defended his MSc thesis on the point set registration in 2018. After that, he joined Intel as an intern and then as a Deep Learning Research Engineer. Dmitrii contributed to the deep learning single image super-resolution and biomedical image segmentation algorithms to OpenVINO. As of 2019, he is a Ph.D. student in the Medical Imaging program of the Medical University of Vienna.
Dmitrii is focusing on Medical Image Analysis and Computer Vision. His research topics include image segmentation, image registration, longitudinal prediction, implicit deep learning models, with applications to healthcare.
Research Interests:
- Medical image analysis
- Computer Vision
- Deep Learning
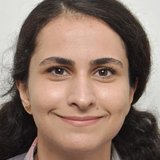
Marzieh Oghbaie, MSc
Computational Imaging Research | PhD StudentPhone: +43 1 40400 66145
Email: marzieh.oghbaie@meduniwien.ac.at
Christian Doppler Laboratory for Artificial Intelligence in Retina
Department of Ophthalmology and Optometry
Medical University of Vienna
Spitalgasse 23
1090 Vienna, Austria
Office: BT72, 17. floor, room 72.17.15
Marzieh obtained her Bsc degree in hardware engineering from K.N. Toosi University of Tehran, Iran(2015). For her master thesis, she worked on text document analysis at Imam Khomeini International University of Qazvin, Iran(2019).
After spending a year as a machine learning researcher and developer at Institute for Research in Fundamental Sciences(IPM), Tehran, Iran, she started her career as an Artificial Intelligence specialist at an e-kyc company in Iran(2019). As a computer vision engineer, she mostly worked on developing video-based models to decipher silent video (lip reading) and low-resource models for image classification.
From August 2022, she has joined Christian Doppler Lab for Artificial Intelligence in Retina, working on OCT image classification. Her main interests include medical image analysis, metric learning, and explainable artificial intelligence.
Research Interests:
- Medical Image Analysis
- Computer Vision
- Deep Learning
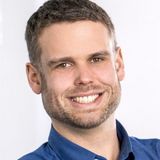
Botond Fazekas, MSc
Computational Imaging Research | PhD StudentPhone: +43 1 40400 66144
Email: botond.fazekas@meduniwien.ac.at
Christian Doppler Laboratory for Artificial Intelligence in Retina
Department of Ophthalmology and Optometry
Medical University of Vienna
Spitalgasse 23
1090 Vienna, Austria
Office: BT72, 17. floor, 72.17.14
Botond Fazekas obtained his BSc in Software Engineering and MSc in Computational Intelligence from the Vienna University of Technology. During and after his graduation he worked for several years as a freelancer contractor for different Austrian and German companies leading large-scale software development projects as a software architect, besides working on research projects focusing on predictive maintenance for locomotives and high-speed computer vision solutions for train pantograph certification and safety evaluation. He joined the research group as a research engineer in 2020 to pursue an academic career and he started his PhD studies in 2021 under the supervision of Hrvoje Bogunović.
Research Interests:
- Medical Image Computing
- Machine Learning interpretability
Selected Publications:
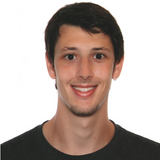
José Morano Sánchez, MSc
Computational Imaging Research | PhD StudentPhone: +43 1 40400 66146
Email: jose.moranosanchez@meduniwien.ac.at
Christian Doppler Laboratory for Artificial Intelligence in Retina
Department of Ophthalmology and Optometry
Medical University of Vienna
Spitalgasse 23
1090 Vienna, Austria
Office: BT72, 17. floor, room 72.17.16
José Morano received his BSc degree in Computer Science in 2019 from the University of A Coruña, Spain. In the same university, he also received his MSc degree in Computer Science in 2022. From 2020 to 2022 he worked as a researcher in the Center for Research in Information and Communication Technologies (CITIC) of A Coruña, Spain. His research work during this period was focused on developing deep learning-based approaches for the automatic analysis of retinal images. In October 2022, José joined the Christian Doppler Laboratory for Artificial Intelligence in Retina of the Medical University of Vienna, where he is currently pursuing his PhD in Medical Imaging.
Research Interests:
- Medical image analysis
- Deep learning
- Computer vision
Markus Unterdechler, BSc
Computational Imaging Research | MSc StudentPhone: +43 1 40400 66144
Email: markus.unterdechler@meduniwien.ac.at
Christian Doppler Laboratory for Artificial Intelligence in Retina
Department of Ophthalmology and Optometry
Medical University of Vienna
Spitalgasse 23
1090 Vienna, Austria
Office: BT72, 17. floor, room 72.17.14
Markus received his bachelor’s degree in computer science from JKU Linz, where he did his thesis on Deep Reinforcement Learning Assisted Monte Carlo Tree Search. Before his studies, he worked as a software engineer and programmed automation software for glass production. During his time at JKU Linz, Markus also worked for the LIT Cyber-Physical Systems Lab, where he analyzed Cyber-Physical Production Systems using Machine Learning, with a focus on Graph Neural Networks.
Markus is currently completing a master’s degree in artificial intelligence and is doing his thesis at the Christian Doppler Laboratory for Artificial Intelligence in Retina
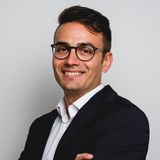
Georg Faustmann, MSc
Software DevelopmentPhone: +43 1 40400 66173
Email: georg.faustmann@meduniwien.ac.at
Christian Doppler Laboratory for Artificial Intelligence in Retina
Department of Ophthalmology and Optometry
Medical University of Vienna
Spitalgasse 23
1090 Vienna, Austria
Office: BT72, 16. floor, room 72.16.13
Georg Faustmann obtained his MSc in logic and computation (computer science) at the TU Wien. His thesis was about optimizing production processes in the industry via machine learning. This thesis was done at the Christian Doppler Laboratory for Artificial Intelligence and Optimization for Planning and Scheduling. Before his studies, he programmed microcontrollers for entrance systems in trains. During his time at the university, he worked at Google Summer of Code and Siemens on a project about smart grids and as a teaching assistant for a functional programming course. As a research engineer at the Christian Doppler Laboratory for Artificial Intelligence in Retina, he integrates state-of-the-art research into applications used by medical staff.
Interested in:
- Full-stack web development
- Supervised Machine Learning
- Functional programming
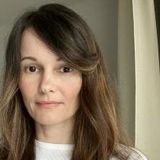
Jasenka Palavric
Reading TeamChristian Doppler Laboratory for Artificial Intelligence in Retina
Department of Ophthalmology and Optometry
Medical University of Vienna
Spitalgasse 23
1090 Vienna, Austria
Office: AKH building (BT 10, 8. floor, room 08.H2.01)
Jasenka graduated from the Business School in Vienna in 1999. Subsequently she has worked as an assistant in the office support area, fashion industry and in an event agency. After parental leave, seeking new challenges, she joined the Optima Reading team in September 2013, she also continues with this work in the Christian Doppler Laboratory for Artificial Intelligence in Retina.
Philip Scheschy
Reading TeamChristian Doppler Laboratory for Artificial Intelligence in Retina
Department of Ophthalmology and Optometry
Medical University of Vienna
Spitalgasse 23
1090 Vienna, Austria
Office: AKH building (BT 10, 8. floor, room 08.H2.01)
Laetitia Hinterhuber
Reading TeamChristian Doppler Laboratory for Artificial Intelligence in Retina
Department of Ophthalmology and Optometry
Medical University of Vienna
Spitalgasse 23
1090 Vienna, Austria
Office: AKH building (BT 10, 8. floor, room 08.H2.01)
Former Team Members
Publications
Projective Skip-Connections for Segmentation Along a Subset of Dimensions in Retinal OCT
Lachinov D, Seeböck P, Mai J, Goldbach F, Schmidt-Erfurth U, Bogunovic H
2021 Medical Image Computing and Computer Assisted Intervention – MICCAI 2021
Preprint link
Deep Dirichlet uncertainty for unsupervised out-of-distribution detection of eye fundus photographs in glaucoma screening
Araujo T, Aresta G, and Bogunovic H
2022 Proc. IEEE International Symposium on Biomedical Imaging Challenges (ISBIC), pp. 1-5
Preprint link
ADAM Challenge: Detecting Age-related Macular Degeneration from Fundus Images
Fang H, Li F, Fu H, Sun X, Cao X, Lin F, Son J, Kim S, Quellec G, Matta S, Shankaranarayana S, Chen Y-T, Wang C-H, Shah NA, Lee C-Y, Hsu C-C, Xie H, Lei B, Baid U, Innani S, Dang K, Shi W, Kamble R, Singhal N, Wang C-W, Lo S-C, Orlando JI, Bogunovic H, Zhang X, Xu Y
2022 IEEE Transactions on Medical Imaging
SD-LayerNet: Semi-supervised retinal layer segmentation in OCT using disentangled representation with anatomical priors
Fazekas B, Aresta G, Lachinov D, Riedl S, Mai J, Schmidt-Erfurth U, and Bogunovic H
2022 Proc. International Conference on Medical Image Computing and Computer-Assisted Intervention (MICCAI), Lecture Notes in Computer Science, In Press
Preprint link
AI-based monitoring of retinal fluid in disease activity and under therapy
Schmidt-Erfurth U, Reiter GS, Riedl S, Seeböck P, Vogl WD, Blodi BA, Domalpally A, Fawzi A, Jia Y, Sarraf D, Bogunović H.
2022 Progress in Retinal and Eye Research
Quantitative assessment of depolarization by the retinal pigment epithelium in healthy and glaucoma subjects measured over a large field of view
Motschi AR, Schwarzhans F, Desissaire S, Steiner S, Bogunović H, Roberts PK, Vass C, Hitzenberger CK, Pircher M
2022 PLoS ONE
Deep survival modeling of longitudinal retinal OCT volumes for predicting the onset of atrophy in patients with intermediate AMD
Rivail A, Vogl WD, Riedl S, Grechenig C, Coulibaly LM, Reiter GS, Guymer RH, Wu Z, Schmidt-Erfurth U, Bogunovic H
2023 Biomedical Optics Express
Automated inter-device 3D OCT image registration using deep learning and retinal layer segmentation
Rivas-Villar D, Motschi AR, Pircher M, Hitzenberger CK, Schranz M, Roberts PK, Schmidt-Erfurth U, Bogunović H
2023 Biomedical Optics Express
Characteristics of Henle’s fiber layer in healthy and glaucoma eyes assessed by polarization-sensitive optical coherence tomography
Motschi AR, Schwarzhans F, Desissaire S, Steiner S, Bogunović H, Roberts PK, Vass C, Hitzenberger CK, Pircher M
2023 Biomed Opt Express
Clinical validation for automated geographic atrophy monitoring on OCT under complement inhibitory treatment
Mai J, Lachinov D, Riedl S, Reiter GS, Vogl WD, Bogunovic H, Schmidt-Erfurth U
2023 Scientific Reports
Segmentation of Bruch's Membrane in Retinal OCT With AMD Using Anatomical Priors and Uncertainty Quantification
Fazekas B, Lachinov D, Aresta G, Mai J, Schmidt-Erfurth U, Bogunovic H
2023 IEEE Journal of Biomedical and Health Informatics
Few-shot out-of-distribution detection for automated screening in retinal OCT images using deep learning
Araújo T, Aresta G, Schmidt-Erfurth U, Bogunović H
2023 Scientific Reports
GAMMA challenge: Glaucoma grAding from Multi-Modality imAges
Wu J, Fang H, Li F, Fu H, Lin F, Li J, Huang Y, Yu Q, Song S, Xu X, Xu Y, Wang W, Wang L, Lu S, Li H, Huang S, Lu Z, Ou C, Wei X, Liu B, Kobbi R, Tang X, Lin L, Zhou Q, Hu Q, Bogunović H, Orlando JI, Zhang X, Xu Y
2023 Medical Image Analysis
Self-supervised Learning via Inter-modal Reconstruction and Feature Projection Networks for Label-Efficient 3D-to-2D Segmentation
Morano J, Aresta, G, Lachinov D, Mai J, Schmidt-Erfurth U, Bogunović H
2023 MICCAI in Lecture Notes in Computer Science
Transformer-Based End-to-End Classification of Variable-Length Volumetric Data
Oghbaie M, Araújo T, Emre T, Schmidt-Erfurth U, Bogunović H
2023 MICCAI in Lecture Notes in Computer Science
Adapting Segment Anything Model (SAM) for Retinal OCT
Fazekas B, Morano J, Lachinov D, Aresta G, Bogunović H
2023 OMIA in Lecture Notes in Computer Science
Vacant positions
Funding
Christian Doppler Laboratory for Artificial Intelligence in Retina – “CD AIR” is supported by the Christian Doppler Research Association in collaboration with Heidelberg Engineering GmbH.
In Christian Doppler Laboratories, application-oriented basic research is pursued at a high level, and expert scientists cooperate with innovative companies. The Christian Doppler Research Association is an international best practice example for promoting this collaboration.
Christian Doppler Laboratories are financed jointly by the public purse and the participating companies. The most important public sponsor is the Federal Ministry for Digital and Economic Affairs (BMDW).
Contact and address
Christian Doppler Laboratory for Artificial Intelligence in Retina
Department of Ophthalmology and Optometry
Medical University of Vienna
Spitalgasse 23
1090 Vienna, Austria
Phone: +43 1 40400 73419
Links
Affiliates
Imprint
Reseponsible for contents:
Hrvoje Bogunovic, PhD
Department of Ophthalmology
Medical University of Vienna
Währinger Guertel 18-20
1090 Vienna