An international research project funded by the 2021 ERAPerMed call for “MULTIDISCIPLINARY RESEARCH PROJECTS ON PERSONALISED MEDICINE – DEVELOPMENT OF CLINICAL SUPPORT TOOLS FOR PERSONALISED MEDICINE IMPLEMENTATION” (ERAPERMED2021-324_PETictCAC)
Whole-body PET imaging systems enable the non-invasive observation and quantification of molecular processes in multiple organs across the entire body. Although these imaging systems support improved patient management, prior data analysis mandates computational tools for efficiency and efficacy. In fact, state-of-the-art patient management, including bespoke imaging tests, requires the analysis of increasingly large and complex datasets. This brings forward the development of artificial intelligence (AI)-based decision support functionalities for a variety of clinical scenarios.
This proposal aims to establish and validate a high-throughput computational framework that exploits AI to support an automated analysis of metabolic interactions between organs based on whole-body [18F]FDG-PET imaging. However, for a wider clinical adoption of such concepts, repeatability of proof-of-concept and lack of effective means to ‘explain’ the inherent decision-making in AI tools must be addressed.
Thus, this project focuses on cancer-associated cachexia (CAC) to analyze pathological aberrations in homeostatic networks of human subjects. CAC is characterized by systemic inflammation affecting different tissues, which impairs the regulation of energy homeostasis. Treatment of CAC focuses mainly on the compensation of weight loss. However, total parenteral nutrition fails to provide sustained clinical benefits. Therefore, novel approaches to diagnose and prognosticate CAC are required for a better patient management.
We aim to develop an AI-supported clinical decision support tool based on whole-body FDG-PET imaging data for the early detection of CAC in lung cancer patients. Incorporating ethical consideration during the design of this clinical tool provides an important added value to ensure regulatory acceptance and clinical roll-out of potential solutions to the bespoke diagnostic dilemma beyond the participating centres.
Project synopsis
Unintentional weight loss is common in patients suffering from advanced cancer. This condition has long been recognised as a frequent and life-threatening complication of many malignancies, but research has only recently begun to uncover its molecular basis. Clinicians refer to cancer associated cachexia (CAC) once weight loss exceeds 5% over 6 months. Therapeutic strategies to revert weight loss after it commenced are ineffective, possibly because they are initiated too late or tackle the wrong pathway. The project explores novel approaches to diagnose CAC before weight loss occurs to implement therapeutic interventions earlier.
We propose to develop a computational framework that uses artificial intelligence (AI) to support an automated analysis of interactions between organs that we can visualise and quantify non-invasively using positron emission tomography (PET). PET imaging of glucose uptake ([18F]FDG-PET) reveals the metabolic connectivity between organs and can conceivably detect the mobilisation of resources in fat and muscle tissue before weight loss weakens the patient. If successful, our computational framework will be developed into a clinical decision-making tool integrating ethical and legal considerations. This tool will determine individual patient risk to develop cachexia and, thus, support personalised therapeutic interventions.
For updates on our research activities towards this objective, please follow us on LinekdIn.
Project Team and Centres
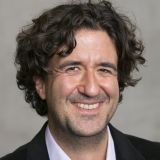
Thomas Beyer, PhD, MBA
CoordinatorMedical University of Vienna, Austria
Leipzig Team
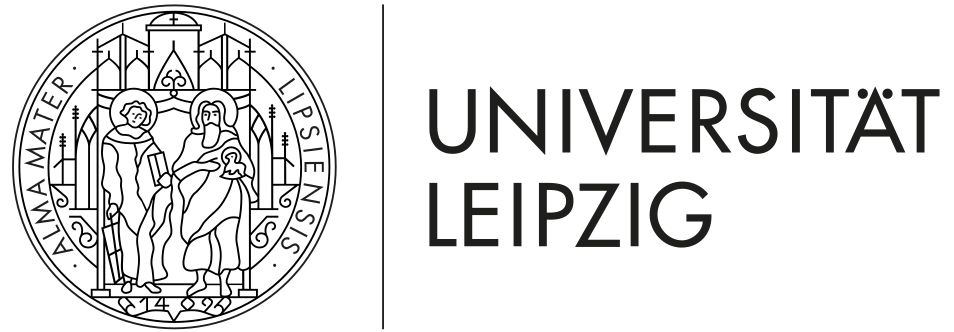
- Osama Sabri, MD
- Swen Hesse, MD
- Armin Frille, MD
- Hubert Wirtz, MD
- Anke Tönjes, MD
- Lukas Hofmann
Funders
The LuCaPET consortium applied successfully for funding in the ERA PerMed Joint Transnational Call 2021 for “Multidisciplinary research projects on personalised medicine – development of clinical support tools for personalised medicine implementation” and acknowledges funding through the Austrian Science Fund, Regione Toscana, Innovation Fund Denmark and Saxon State Ministry for Higher Education, Research and the Arts.