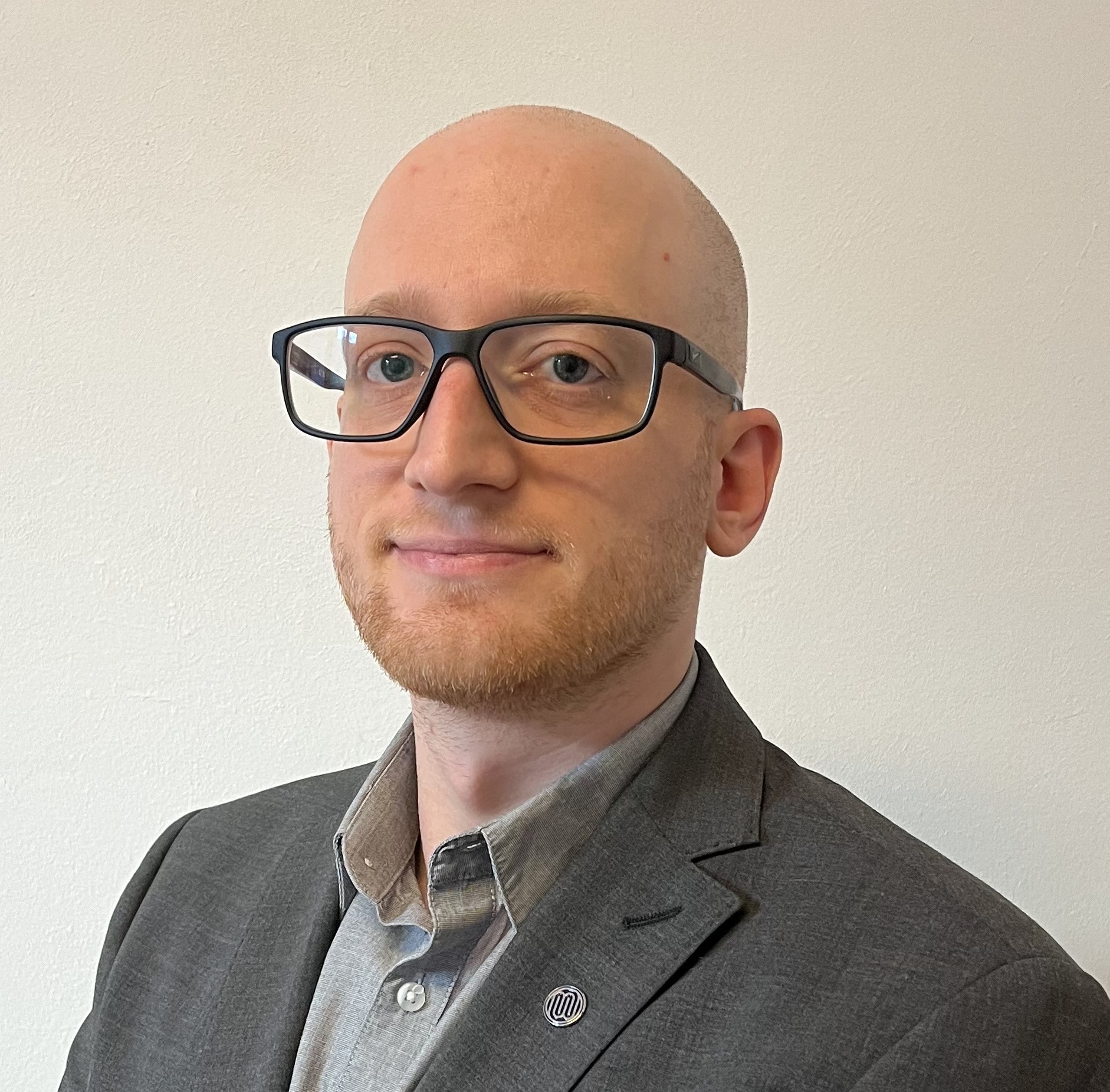
Department of Biomedical Imaging and Image-guided Therapy (Division of Nuclear Medicine)
Position: Research Associate (Postdoc)
ORCID: 0000-0002-4409-8324
T +43 1 40400 72350
clemens.spielvogel@muv.ac.at
Keywords
Amyloidosis; Artificial Intelligence; Automatic Data Processing; Cardiac Imaging Techniques; Computational Biology; Data Mining; Decision Making, Computer-Assisted; Electronic Health Records; Image Interpretation, Computer-Assisted; Image Processing, Computer-Assisted; Medical Informatics; Nuclear Medicine; Pattern Recognition, Automated
Research group(s)
- Computational Nuclear Medicine
Head:
Members:
Research interests
Discovery, development, and clinical assessment of novel biomarkers and clinical decision support systems.
Focus areas include nuclear medicine, oncological, and cardiovascular diseases.
Techniques, methods & infrastructure
- Machine learning
- Vision-based deep learning
- Explainable artificial intelligence
- Cardiovascular imaging
- Quantitative imaging markers
- Imaging / non-imaging data integration
- Opportunistic risk markers
Selected publications
- Spielvogel, C.P. et al. (2024) ‘Diagnosis and prognosis of abnormal cardiac scintigraphy uptake suggestive of cardiac amyloidosis using artificial intelligence: a retrospective, international, multicentre, cross-tracer development and validation study’, The Lancet Digital Health, 6(4), pp. e251–e260. Available at: https://doi.org/10.1016/s2589-7500(23)00265-0.
- Haberl, D. et al. (2025) ‘Generative artificial intelligence enables the generation of bone scintigraphy images and improves generalization of deep learning models in data-constrained environments’, European Journal of Nuclear Medicine and Molecular Imaging, 52(7), pp. 2355–2368. Available at: https://doi.org/10.1007/s00259-025-07091-8.
- Spielvogel, C.P. et al. (2024) ‘Preoperative detection of extraprostatic tumor extension in patients with primary prostate cancer utilizing [68Ga]Ga-PSMA-11 PET/MRI’, Insights Into Imaging. Available at: https://doi.org/10.1186/s13244-024-01876-5.
- Spielvogel, C.P. et al. (2022) ‘Radiogenomic markers enable risk stratification and inference of mutational pathway states in head and neck cancer’, European Journal of Nuclear Medicine and Molecular Imaging, 50(2), pp. 546–558. Available at: https://doi.org/10.1007/s00259-022-05973-9.
- Spielvogel, C.P. et al. (2025) ‘Artificial intelligence-enabled opportunistic identification of immune checkpoint inhibitor-related adverse events using [18F]FDG PET/CT’, European Journal of Nuclear Medicine and Molecular Imaging [Preprint]. Available at: https://doi.org/10.1007/s00259-025-07364-2.