Kurzer Teaser zur Organisation der Posterausstellung und den Guided Sessions. Einladung, die Verfasser mit Fragen direkt zu kontaktieren oder die Guided Session zu besuchen.
Hier könnte ein Abstract zum Topic der Postersession sein.
Außerdem eine Info, wer die Postersession leitet und wo sie stattfindet. Wenn die Session online stattfindet und frei zugänglich ist, sollte sie hier auch gleich verlinkt sein.
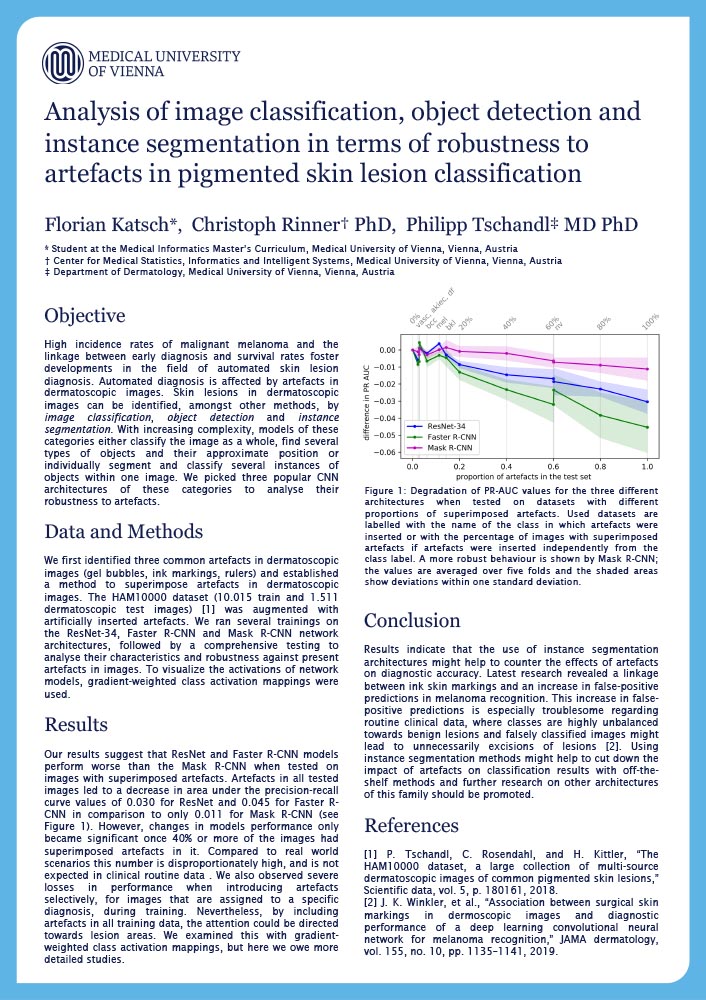
Florian Katsch
Student at the Medical Informatics Master’s Curriculum, Medical University of Vienna
Introduction
High incidence rates of malignant melanoma and the linkage between early diagnosis and survival rates foster developments in the field of automated skin lesion diagnosis. Automated diagnosis is affected by artefacts in dermatoscopic images. These artefacts in images can be identified amongst other methods by image classification, object detection and instance segmentation. We picked three popular CNN architectures of these categories to analyse their robustness to artefacts.
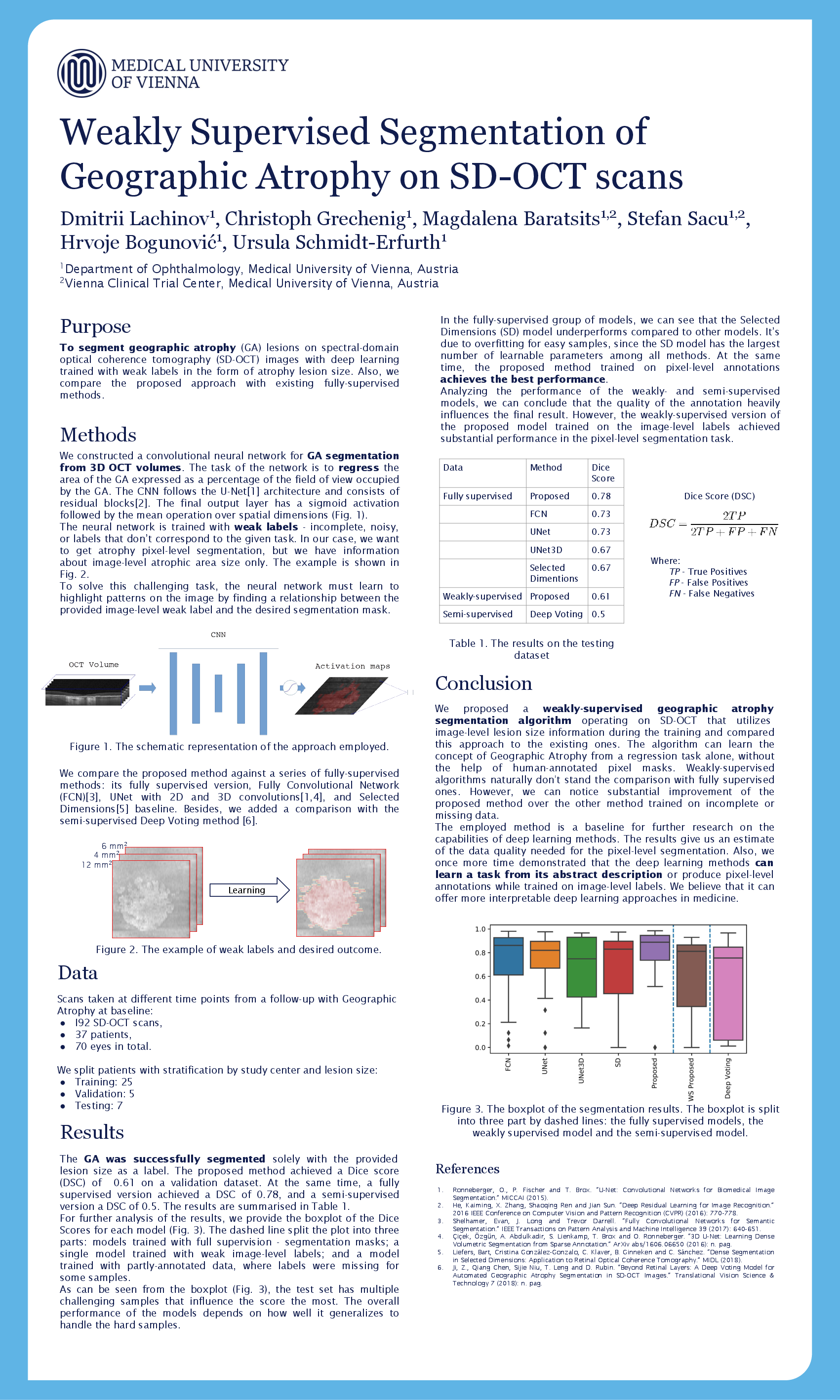
Dmitrii Lachinov
Department of Ophthalmology, Medical University of Vienna
Introduction
To segment geographic atrophy (GA) lesions on spectral-domain optical coherence tomography (SD-OCT) images with deep learning trained with weak labels in the form of atrophy lesion size. In addition, we compare the proposed approach with existing fully pixel-level supervised methods.
We constructed a convolutional neural network for GA segmentation from 3D OCT volumes. The task of the network is to regress the area of the GA expressed as a percentage of the field of view occupied by the GA. The CNN follows the U-Net architecture and consists of residual blocks. The final output layer has a sigmoid activation followed by the mean operation. The neural network learns to highlight patterns on the image by finding a relationship between the provided weak label and the desired segmentation mask. We compare the performance of our method against the supervised version of our algorithm and against the semi-supervised deep-voting model.