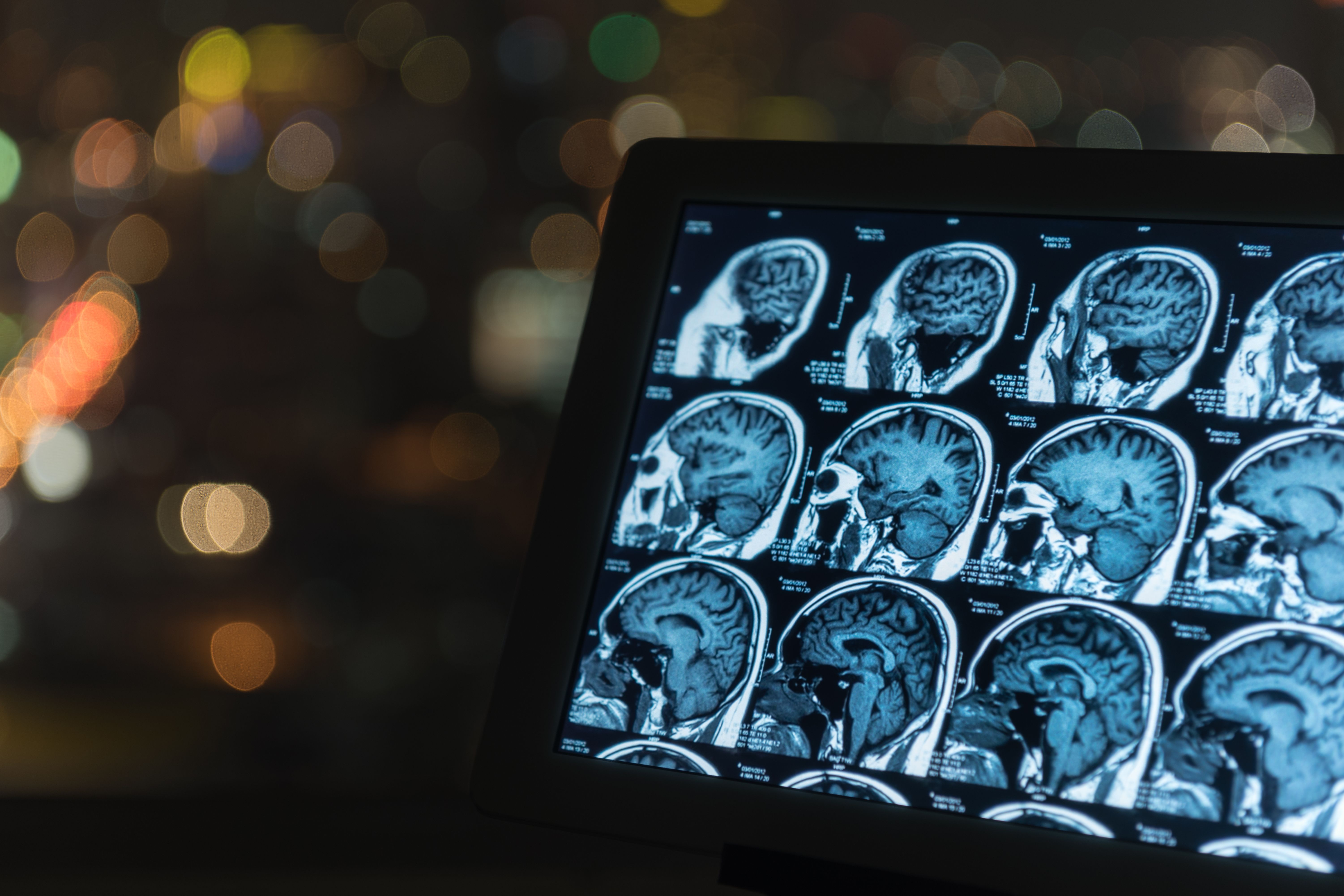
(Vienna, 28 September 2021) Just because models in the field of machine learning in medicine work, that does not mean that it will stay that way. During their deployment to support diagnosis, diagnostic technology - their source of data - changes and they fall out of date. In a paper published today in the journal Nature Communications, researchers from MedUni Vienna and colleagues from the USA present a new method by which models can continually learn while the world around them changes.
Models in the area of machine learning deployed to clinical routine use diagnostic imaging to aid diagnosis and prediction of the course of disease for individual patients. During deployment they are faced with the influence of the constant advancement of imaging technologies and diagnostic procedures. What works now can quickly fall out-of-date while new scanners are added to the repertoire and image characteristics change regardless of disease patterns. The accuracy and robustness of the algorithms might deteriorate.
In this work Matthias Perkonigg (Computational Imaging Research Lab, Department of Biomedical Imaging and Image-guided Therapy) and colleagues are presenting a new continual learning approach which enables to accumulate knowledge about image characteristics from a continuous stream of data without losing the ability to analyse data acquired with prior technology. The method uses two strategies for this. During continuous training with imaging data the algorithm keeps a constantly changing dynamic memory to ensure the diversity of training data. On the one hand, it enables new image characteristics to be recognized and integrated, while at the same time keeping the memory diverse. The second strategy automatically recognizes clusters of samples with similar features, so called pseudo-domains. Those clusters might represent clinical centers with similar acquisition equipment or distinct acquisition protocols.
The method can be used in various fields, in this paper its advantages are shown both in cardiological imaging with MRI and in lung imaging with CT.
Service: Nature Communications
Matthias Perkonigg, Johannes Hofmanninger, Christian Herold, James Brink, Oleg Pianykh, Helmut Prosch, and Georg Langs. Dynamic memory to alleviate catastrophic forgetting in continual learning with medical imaging. (2021) Nature Communications.
https://doi.org/10.1038/s41467-021-25858-z
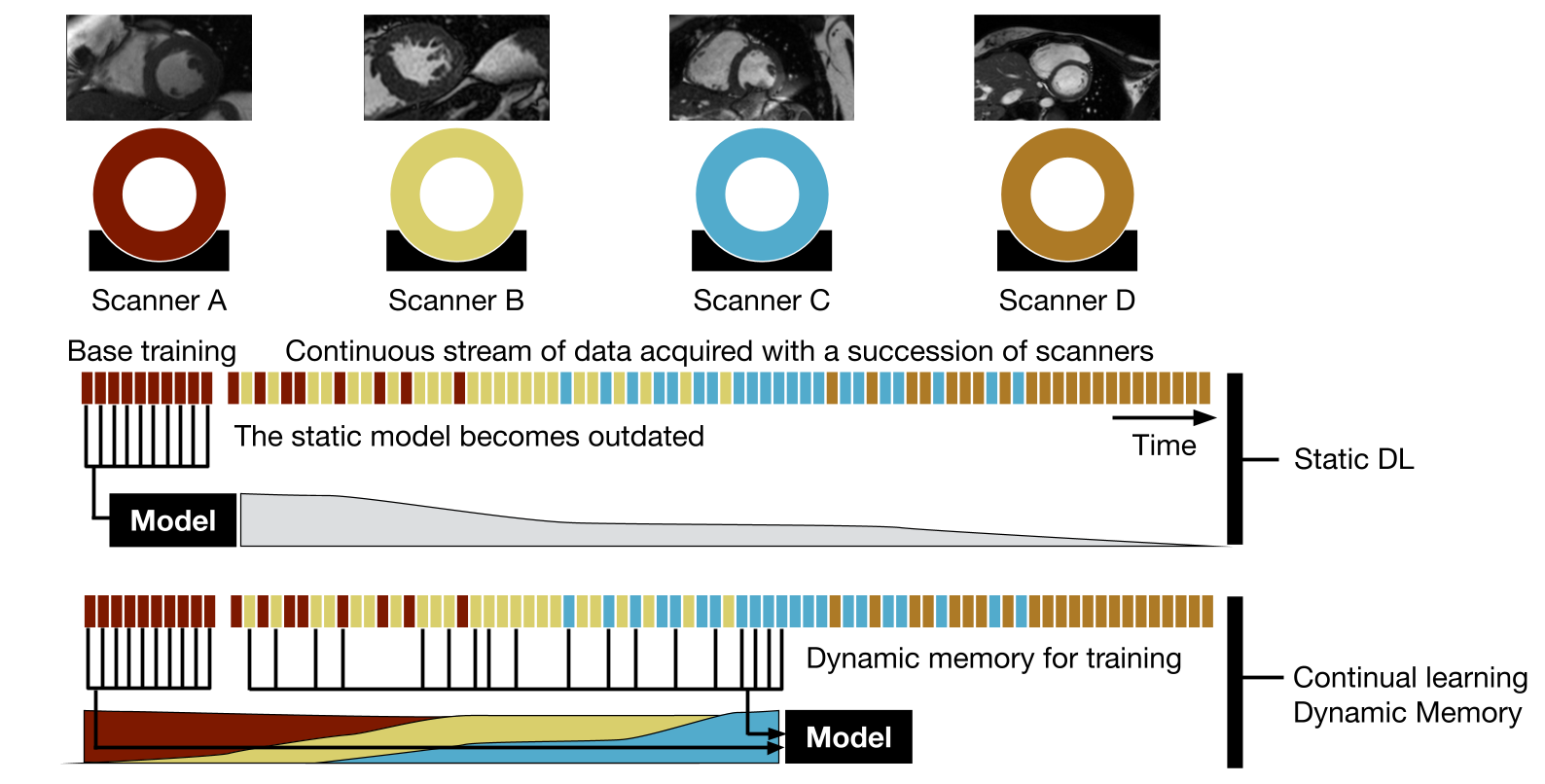
If left static, machine learning models fall out of date as imaging technology advances. Continual learning adapts the learning to an expanding diversity of scanners.