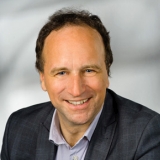
Center for Medical Data Science (Institute of Artificial Intelligence)
Position: Associate Professor
ORCID: 0000-0002-3181-2576
T +43 1 40160 36316
georg.dorffner@meduniwien.ac.at
Keywords
Artificial Intelligence; Electroencephalography; Medical Informatics; Neural Networks (Computer); Pattern Recognition, Automated; Signal Processing, Computer-Assisted; Sleep Stages; Support Vector Machines
Research interests
My main research focus is on applying state-of-the-art methods from digital signal processing and machine learning to the development of diagnostic support in various clinical fields. One strong focus in recent years has been on recognizing patterns in electroencephalograms (EEG), and scoring such data in sleep medicine. An example is the automated staging of sleep based on EEG and other biosignals such as electrooculography (EOG) and electromyography (EMG) according to international standards. Another focus is the use of machine learning methods for predicting diseases or their outcome, as well as modelling their uncertainty toward more robustness.
Techniques, methods & infrastructure
Machine learning, neural networks, Gaussian mixture models, Bayesian learning, time series processing, signal processing, Matlab
Grants
- DS4Health - International Master's Program Empowering Healthcare through Digital Technology (2023)
Source of Funding: EU, DIGITAL Europe - European Health and Digital Executive Agency (HADEA)
Principal Investigator - DS4Health - International Master's Program Empowering Healthcare through Digital Technology (2023)
Source of Funding: Medical University of Vienna, DIGITAL Europe, EU Commission, European Health and Digital Executive Agency (HADEA)
Principal Investigator - Digital Skills, Knowledge and Communication for students of medicine (2020)
Source of Funding: Medical University of Vienna, Digital Transformation, Federal Ministry of Education, Science and Research
Principal Investigator - Deep learning for improved nuclei segmentation and knowledge transfer methods in microscopic images (2019)
Source of Funding: FFG (Austrian Research Promotion Agency), Bridge Young Scientists
Principal Investigator - Imaging neuronal circuits of the preforntal cortex during a gambling task (2015)
Source of Funding: WWTF (Vienna Science and Technology Fund), Life Sciences
Principal Investigator
Selected publications
- Mahbod, A. et al. (2024) ‘Improving generalization capability of deep learning-based nuclei instance segmentation by non-deterministic train time and deterministic test time stain normalization’, Computational and Structural Biotechnology Journal, 23, pp. 669–678. Available at: https://doi.org/10.1016/j.csbj.2023.12.042.
- Brandmayr, G. et al. (2022) ‘Relational local electroencephalography representations for sleep scoring’, Neural Networks, 154, pp. 310–322. Available at: https://doi.org/10.1016/j.neunet.2022.07.020.
- Agibetov, A. et al. (2021) ‘Convolutional Neural Networks for Fully Automated Diagnosis of Cardiac Amyloidosis by Cardiac Magnetic Resonance Imaging’, Journal of Personalized Medicine, 11(12), p. 1268. Available at: https://doi.org/10.3390/jpm11121268.
- Wallisch, C. et al. (2021) ‘The roles of predictors in cardiovascular risk models - a question of modeling culture?’, BMC Medical Research Methodology, 21(1). Available at: https://doi.org/10.1186/s12874-021-01487-4.
- Agibetov, A. et al. (2020) ‘Machine Learning Enables Prediction of Cardiac Amyloidosis by Routine Laboratory Parameters: A Proof-of-Concept Study’, Journal of Clinical Medicine, 9(5), p. 1334. Available at: https://doi.org/10.3390/jcm9051334.