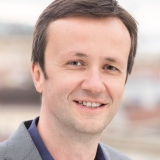
Center for Medical Data Science (Institute of Clinical Biometrics)
Position: Professor
ORCID: 0000-0003-1147-8491
T +43 1 40400 66890
georg.heinze@meduniwien.ac.at
Keywords
Confounding Factors (Epidemiology); Models, Statistical; Prognosis
Research group(s)
- Prognosis research
Research Area: Prognosis research in medicine aims at understanding and improving future outcomes of individuals. We work on aspects of developing, translating and applying statistical methodology in prognosis research. Co-leaders: Daniela Dunkler and Georg Heinze
Members: - Biometrical consulting
Head: Georg Heinze
Research Area: Our biometrical consulting service supports clinical research at the Medical University of Vienna. All biostatistical aspects of study planning, preparation of study protocol, and data analysis can be discussed. See our website for details.
Members:
Research interests
My work is devoted to statistical modeling for prognosis research, in particular to all aspects of developing, translating and applying statistical methodology. Prognosis research in medicine aims at understanding and improving future outcomes of patients (Hemingway BMJ 2013). I develop, translate and apply biostatistical methods with observational data (see refs. 1, 2, 3).
Develop:
Our review of variable selection (ref. 4) has been classified by Clarivate Analytics Web of Science as ‘Hot Paper’, placed in the top 0.1% of papers by citation intensity in the academic field of Mathematics. I have developed methods for statistical modeling with rare outcome events and sparse data (e.g. ref 5).
Translate:
The STRATOS initiative (www.stratos-initiative.org), in which I serve as member of the steering group and chair of a topic group, aims at developing guidance documents that translate statistical knowledge to the research community at large. Moreover, I have published a series of educational papers for the medical research community.
Apply:
I also apply state-of-the-art methodology in clinical epidemiology and health services research and I have published several observational studies as first author. I contributed to more than 300 collaborative papers.
Techniques, methods & infrastructure
In prognosis research, we focus on statistical modeling for data description, prediction and causal inference. In several research projects, we have in particular investigated the Firth penalty (Jeffreys prior) as a solution to the problem of non-existence of regression coefficients estimated by maximum likelihood in various risk models. Other research has focused on algorithmic variable selection methods, on analyses of high-dimensional (omics) data or on the optimization of prediction models.
In evaluating new methodology, we use simulation studies, by which we can learn how methods perform under various conditions. In our simulation studies, we always try to define scenarios that are likely to be encountered in real-life data analysis. While in single-data set analyses the underlying population is usually unknown, simulation studies have the advantage that the population properties are defined by the experimentator, enabling the generalizability of results.
Grants
- TOPSTATS-MICRODATA (2024)
Source of Funding: OeAW (Austrian Academy of Sciences), Data:Research:Austria
Principal Investigator - RIPOSTE-BD (2023)
Source of Funding: FWF (Austrian Science Fund), Standalone-Projects
Principal Investigator - SAMBA (2020)
Source of Funding: FWF (Austrian Science Fund), International Projects
Principal Investigator - SCOUT - Supporting Causal Conclusions from Observational Survival Studies (2018)
Source of Funding: EU, H2020-MSCA-IF-2017
Coordinator of the collaborative project - CaReSyAn - Combatting the CardioRenal Syndrome: towards an integrative analysis to reduce cardiovascular burden in chronic kidney disease (2017)
Source of Funding: EU, ITN
Principal Investigator - Predicting rare events more accurately (PREMA) (2015)
Source of Funding: FWF (Austrian Science Fund), Joint projects
Principal Investigator
Selected publications
- Collins, G.S. et al. (2024) ‘TRIPOD+AI statement: updated guidance for reporting clinical prediction models that use regression or machine learning methods’, BMJ, p. e078378. Available at: https://doi.org/10.1136/bmj-2023-078378.
- Kyrle PA, Eischer L, Sinkovec H, Gressenberger P, Gary T, Brodmann M, Heinze G, Eichinger S. (2023) ‘The Vienna Prediction Model for identifying patients at low risk of recurrent venous thromboembolism: a prospective cohort study’, European Heart Journal, 45(1), pp. 45–53. Available at: https://doi.org/10.1093/eurheartj/ehad618.
- Heinze G, Boulesteix AL, Kammer M, Morris T, White I, for the Simulation Panel of the STRATOS initiative. (2024) ‘Phases of methodological research in biostatistics—Building the evidence base for new methods’, Biometrical Journal, 66(1). Available at: https://doi.org/10.1002/bimj.202200222.
- Heinze G, Wallisch C, Dunkler D, 2018. Variable selection - A review and recommendations for the practicing statistician. Biometrical Journal 60(3):431-449. Available at: http://dx.doi.org/10.1002/bimj.201700067.
- Heinze G, Schemper M, 2002. A solution to the problem of separation in logistic regression. Statistics in Medicine, 21(16):2409-2419. Available at: http://dx.doi.org/10.1002/sim.1047.