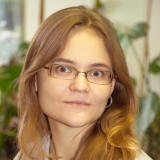
Center for Medical Data Science (Institute of Clinical Biometrics)
Position: Associate Professor
ORCID: 0000-0003-1339-0311
T +43 1 40400 66820
daniela.dunkler@meduniwien.ac.at
Keywords
Biostatistics; Epidemiology; Models, Statistical; Prognosis; Public Health; Regression Analysis; Risk Assessment; Survival Analysis
Research group(s)
- Prognosis research
Research Area: Prognosis research in medicine aims at understanding and improving future outcomes of individuals. We work on aspects of developing, translating and applying statistical methodology in prognosis research. Co-leaders: Daniela Dunkler and Georg Heinze
Members: - Biometrical consulting
Head: Georg Heinze
Research Area: Our biometrical consulting service supports clinical research at the Medical University of Vienna. All biostatistical aspects of study planning, preparation of study protocol, and data analysis can be discussed. See our website for details.
Members:
Research interests
My primary interest is in statistical modeling in etiologic and predictive research. A focus of my methodological research is objective and reproducible variable selection. Obtaining and communicating easily comprehensible effect size measures to support individual treatment decisions or to support public health recommendations is another research focus.
Another area of interest is the application of statistical modeling approaches to the investigation of epidemiological research questions. For example, in our current project, we want to develop methodology to enable long-term risk prediction from short-term big data. In another project, we are currently investigating the amount of variation in an estimate of interest induced by the "garden of forkink path" problem when analyzing observational data using medical and epidemiological case studies.
I take part in the STRATOS initiative (Strengthening Analytical Thinking for Observational Studies).
What I love about my profession? While as a statistician I can develop new methods and algorithms to learn from the data, as a biostatistician & epidemiologist I can apply these methods to extract important patterns and to separate ‘information’ from ‘noise’—in short to make sense of medical and public health data.
Techniques, methods & infrastructure
For routine application of all new methodological developments software implementations in standard statistical software are made publicly available at https://data-science.meduniwien.ac.at/institute/klinische-biometrie/forschung/software/.
Two risk prediction models for incidence or progression of chronic kidney disease are also publicly available at http://www.meduniwien.ac.at/nephrogene/data/riskcalc/renal_v4/renal.html. Our updated Framingham 2008 risk prediction tool for cardiovascular diseases within the next 5 years can be found at https://cvdrisk.shinyapps.io/english/.
To conveniently obtain effect size measures with accompanying 95% confidence intervals for two proportions or two survival rates at a specific time point, our online calculator can be used.
Interesting R packages include coxphw, which implements weighted estimation in Cox regression, and shrink, which estimates global, parameterwise and joint shrinkage factors.
Observational medical research critically depends on good study design, excellent data quality, appropriate statistical methods and accurate interpretation of results. In my course 'How to recognize bad research?' for researchers and consumers of research held at the MedUni Vienna, I give guidance on important statistical issues related to evidence-based medicine.
Grants
- Long-Term Risk Prediction from Short-Term Big Data (2023)
Source of Funding: FWF (Austrian Science Fund), Einzelprojekt
Principal Investigator - Statistical Model Building Strategies for Cardiologic Applications (2020)
Source of Funding: FWF (Austrian Science Fund), International project (Germany DFG - Austria FWF)
Principal Investigator - CVD-Risk ‘Development of an Austrian Risk Prediction Model for the Assessment of the Cardiovascular Risk (2015)
Source of Funding: OeNB (Oesterreichische Nationalbank), Anniversary Fund
Principal Investigator - TOWARDS PRECISE STATISTICAL ANALYSIS PLANS FACILITATING MICRODATA ANALYSES TO ADVANCE HEALTH RESEARCH (2004)
Source of Funding: OeAW (Austrian Academy of Sciences), Data:Research:Austria
Principal Investigator
Selected publications
- Wallisch, C. et al., 2019. External validation of two Framingham cardiovascular risk equations and the Pooled Cohort equations: A nationwide registry analysis. International Journal of Cardiology, 283, pp.165–170. Available at: http://dx.doi.org/10.1016/j.ijcard.2018.11.001.
- Dunkler, D. et al., 2019. To test or to estimate? P‐values versus effect sizes. Transplant International. Available at: http://dx.doi.org/10.1111/tri.13535.
- Dunkler, D. et al., 2014. Augmented Backward Elimination: A Pragmatic and Purposeful Way to Develop Statistical Models J. Olivier, ed. PLoS ONE, 9(11), p.e113677. Available at: http://dx.doi.org/10.1371/journal.pone.0113677.
- Dunkler, D., Sauerbrei, W. & Heinze, G., 2016. Global, Parameterwise and Joint Shrinkage Factor Estimation. J. Stat. Soft., 69(8). Available at: http://dx.doi.org/10.18637/jss.v069.i08.
- Dunkler, D. et al. (2019) ‘To test or to estimate? P ‐values versus effect sizes’, Transplant International, 33(1), pp. 50–55. Available at: http://dx.doi.org/10.1111/tri.13535.