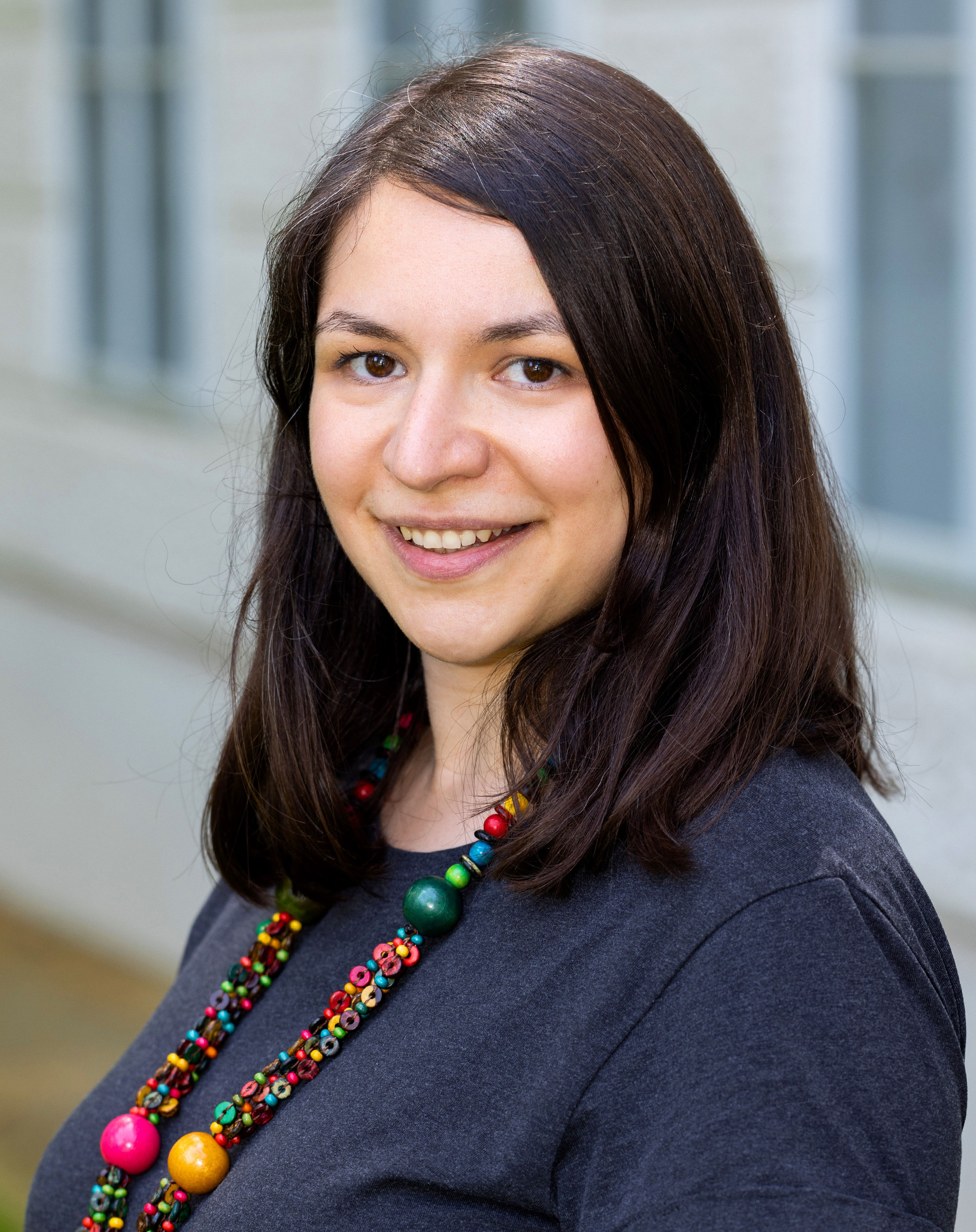
Department of Medicine III (Division of Rheumatology)
Position: Research Associate (Postdoc)
ORCID: 0000-0002-0892-7068
anela.tosevska@meduniwien.ac.at
Keywords
Computational Biology; Data Interpretation, Statistical; Data Mining; Epigenomics; Fibroblasts; Genomics; Rheumatic Diseases; Sequence Analysis; T Cells; Transcriptome
Research group(s)
- Rheumatology Research Lab
Research Area: The main interest of our laboratory is to understand the exact mechanisms that are important for cell stability and identity in T cell-mediated autoimmune diseases such as Systemic Lupus Erythematosus or Rheumatoid Arthritis
Members: - Bioinformatics in Rheumatology Research Group
Head: Anela Tosevska
Research Area: Research area: We are applying computational solutions to contribute to the understanding of inflammatory pathomechanisms and a personalized medical approach, bridging the gap between data, bench and bedside in the rheumatology field. We are continuously looking for highly motivated individuals to join our team.
Members:
Research interests
My research focuses on providing a better insight on the molecular basis of autoimmune rheumatic diseases using computational approaches. I am working closely with wet-lab scientists and clinicians to generate tailored analysis for experimental data and answer complex biological questions in rheumatology. Use of bioinformatic algorithms for integration of high-throughput and experimental data allows us to perform interdisciplinary research to understand drivers of inflammation and develop a more personalized treatment approach. Several projects are currently ongoing which address the specific role of cell-cell interactions and environmental factors on the transcriptome and epigenome of pathogenic cell types. A major focus lies on epigenetic regulation of cell stability under steady state and autoimmune conditions. Other areas of interests are liquid biopsies and cell-free nucleic acids for biomarker development.
Techniques, methods & infrastructure
-
Statistical Programming Languages and Environments: R/Bioconductor, Bash scripting, Python, High Performance Computing: SGE, Slurm;
-
Curation, analysis and interpretation of sequencing data: bulk RNAseq, scRNAseq, BSseq, ChIPseq, ATACseq, WGS;
-
Integration and visualization of multi-omics datasets, including NGS, mass cytometry and proteomics;
-
Statistical analysis of clinical data;
-
Machine Learning: Caret/Scikit-Learn.
Selected publications
- Kugler, M. et al. (2023) ‘Cytokine-directed cellular cross-talk imprints synovial pathotypes in rheumatoid arthritis’, Annals of the Rheumatic Diseases, p. ard-2022-223396. Available at: http://dx.doi.org/10.1136/ard-2022-223396.
- Suto, T. et al. (2022) ‘TNFR2 is critical for TNF-induced rheumatoid arthritis fibroblast-like synoviocyte inflammation’, Rheumatology, 61(11), pp. 4535–4546. Available at: http://dx.doi.org/10.1093/rheumatology/keac124.
- Tosevska, A. et al. (2022) ‘Cell-Free RNA as a Novel Biomarker for Response to Therapy in Head & Neck Cancer’, Frontiers in Oncology, 12. Available at: http://dx.doi.org/10.3389/fonc.2022.869108.
- Tosevska, A. et al. (2022) ‘Integrated analysis of an in vivo model of intra-nasal exposure to instilled air pollutants reveals cell-type specific responses in the placenta’, Scientific Reports, 12(1). Available at: http://dx.doi.org/10.1038/s41598-022-12340-z.
- Farrell, C. et al. (2021) ‘BiSulfite Bolt: A bisulfite sequencing analysis platform’, GigaScience, 10(5). Available at: http://dx.doi.org/10.1093/gigascience/giab033.